Peng Qi
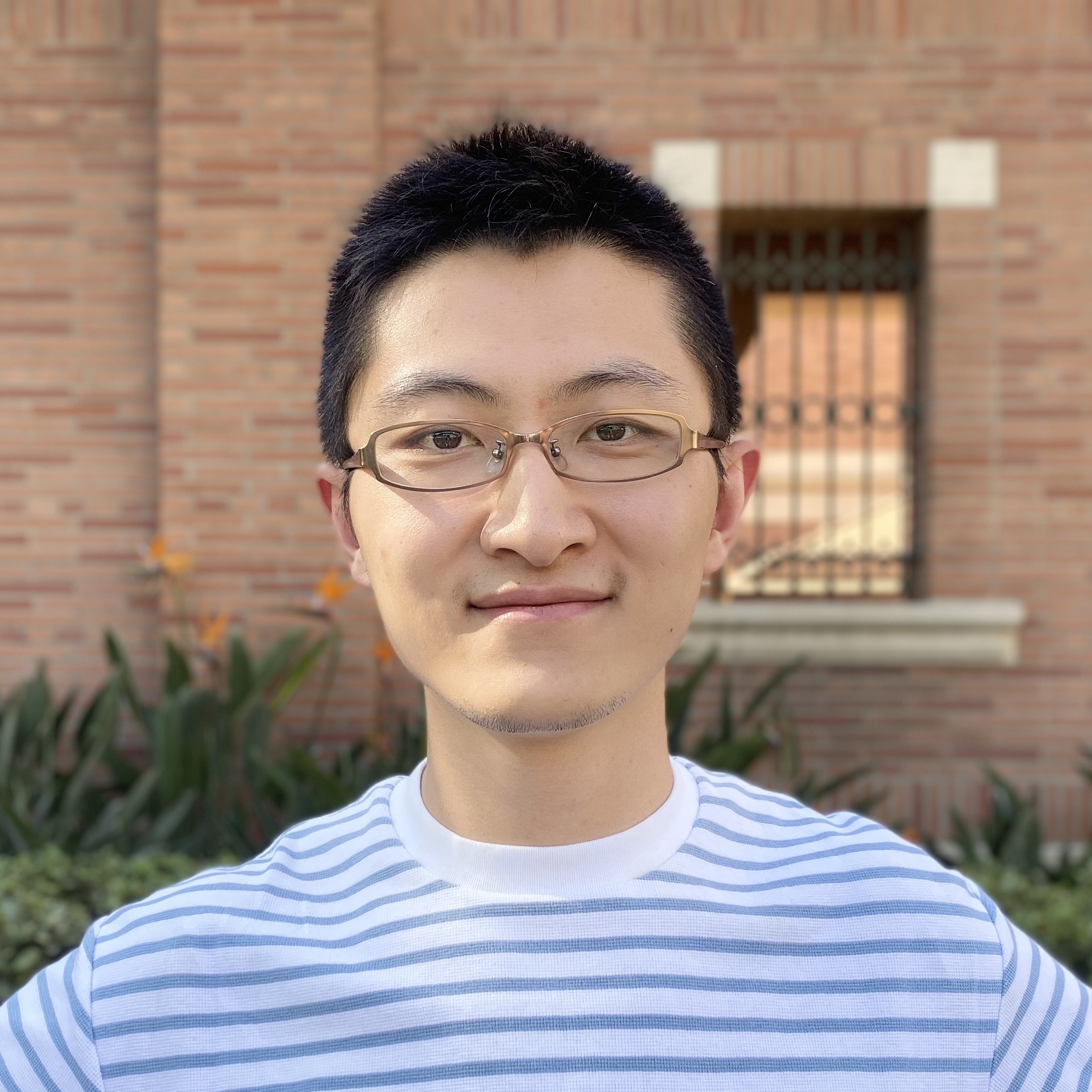
齐鹏
(pinyin: /qí péng/; ipa: /tɕʰǐ pʰə̌ŋ/)
I am an AI researcher working on natural language processing, machine learning, and multimodal agents. I am currently leading research efforts at Orby AI.
My research is driven by the goal of bringing the world’s knowledge to the user’s assistance, which manifests itself in the following directions
- How to effectively organize and use knowledge. This involves tasks like question answering (where I have co-lead the development of some benchmarks for complex reasoning: HotpotQA and BeerQA), information extraction, syntactic analysis for many languages (check out Stanza), etc.
- How to effectively communicate knowledge. This mainly concerns interactive NLP systems such as conversational systems, where I am interested in theory-of-mind reasoning under information asymmetry (e.g., how to ask good questions and how to provide good answers beyond the literal answer), offline-to-online transfer, multi-modal interactions, etc. On the application side, I co-lead the founding research team that launched Amazon Q at Amazon.
- How to leverage interactive knowledge to help users better perform tasks. This mainly concerns multimodal digital agents operating on real-world user interfaces and solving problems on behalf of users. Want to learn more? Consider joining our research team at Orby AI!
In all of these directions, I am also excited to explore data-efficient models and training techniques, model and system explainability, and self-supervised learning techniques that enable us to address these problems.
Before joining Orby, I worked for Amazon Web Services (AWS) as an senior applied scientist, and JD.com AI Research as a senior research scientist before that. I obtained my Ph.D. in Computer Science at Stanford University advised by Prof. Chris Manning, where I was a member of the NLP group and AI Lab. I also obtained two Master’s at Stanford (CS & Statistics), and my Bachelor’s at Tsinghua University.
latest posts
selected publications
- arXiv
- EMNLPDancing in Chains: Reconciling Instruction Following and Faithfulness in Language ModelsIn Empirical Methods in Natural Language Processing (EMNLP), 2024
- EMNLPRAG-QA Arena: Evaluating Domain Robustness for Long-form Retrieval Augmented Question AnsweringIn Empirical Methods in Natural Language Processing (EMNLP), 2024
- EMNLP FindingsTokenization Consistency Matters for Generative Models on Extractive NLP TasksIn Findings of the Association for Computational Linguistics: EMNLP , 2023